Embracing Disruption
DeepSeek – the Growth Equity view
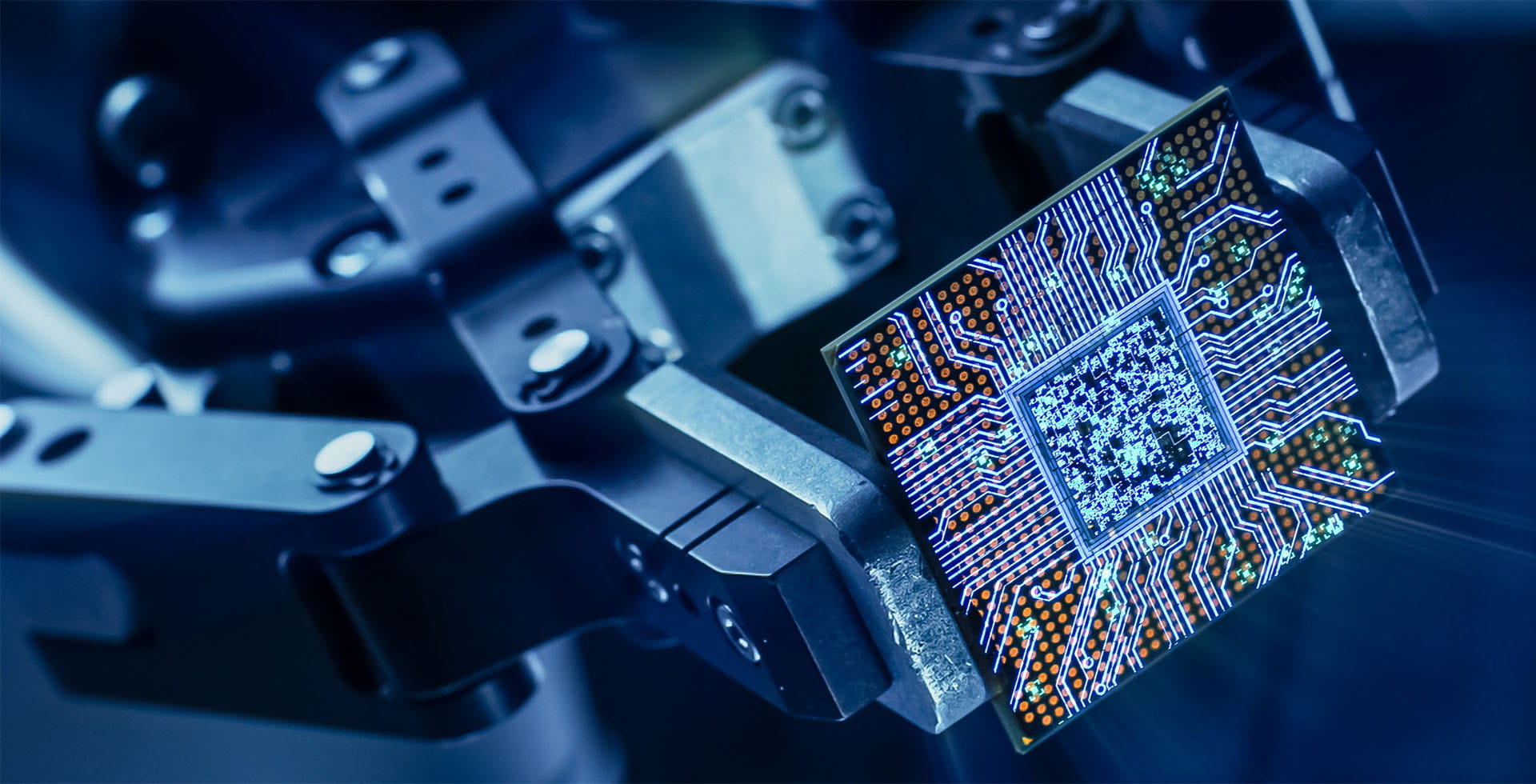
Artificial intelligence (AI) has been one of the dominant themes in the markets for the past year or so (the other being GLP-1 weight loss drugs). Vast amounts of investment have recently been made in AI infrastructure, and suppliers to the sector have been seen by many as the initial winners of this new age. Nvidia, which makes the graphical processing units (GPUs) on which AI models are trained and run, has been the highest profile winner, but other companies have also benefitted significantly, including VAT Group (maker of vacuum valves required in semiconductor manufacturing), Atlas Copco (maker of compressors and vacuum pumps used in semiconductor fabrication) and Amphenol (manufacturer of interconnects and sensors used in data centres).
Until recently, US companies were leading the race for AI model leadership, with ChatGPT from OpenAI (backed by Microsoft), Gemini from Alphabet, and Llama from Meta being seen as the front runners. These companies have invested vast amounts in datacentres and in AI model training to obtain and maintain leadership positions: the training run for Meta’s latest model alone reportedly cost 60-70m USD. At a broader level, the US government has seen AI leadership as a crucial geopolitical advantage, seeking to restrict China’s access to cutting-edge GPUs in order to hinder its rival’s progress.
DeepSeek R1, a new LLM (Large Language Model) released last week, has been seen as a potential gamechanger in the race for AI leadership. This model, released under an open source license by a private Chinese firm, stunned many western observers by generating results comparable with leading open reasoning models from OpenAI for tasks like coding, mathematics and reasoning at around 10% lower cost. This is a remarkable achievement given the restrictions on access to cutting-edge GPUs that Chinese AI researchers operate under. Some western commentators have even referred to this as a ‘Sputnik moment’ for AI.
The source model for DeepSeek R1, called DeepSeek V3, was reportedly trained for just 10% of the cost and in 10% of time as Meta’s Llama 3 model. DeepSeek’s lower costs come from using optimized training and inference techniques, which the engineers were forced to do as a result of US actions preventing Chinese firms from accessing cutting edge GPUs.
However, many investors interpreted the improved computation techniques and low training cost to mean that AI model training might be possible with much lower GPU intensity than previously thought. This has led to concerns about the future revenue opportunities for Nvidia and the GPU supply chain, and has resulted in a (negative) reaction to stocks that have been AI winners over the past 18 months.
More broadly, DeepSeek R1 is intensifying the debate on AI capital expenditure (capex). On the one hand, for some months there have been concerns voiced by investors that the hundreds of billions spent on AI are yet to be effectively monetised, something which might limit future AI investment. On the other hand, major AI users such as Meta (65bn USD of capex guided for 2025) and Microsoft (80bn USD capex) continue to invest vast amounts in the technology and, just last week, AI project Stargate, announced by US president Donald Trump, promised up to 500bn USD capex over four years.
The techniques deployed by DeepSeek appear to represent a step change in the efficiency of model training, which will likely permit a significant reduction in costs. Yet, counterintuitively, this suggests that demand might rise even more. This situation, also known as Jevon’s paradox, suggests big in the cost of a resource can be outweighed by increases in demand brought about by increased adoption. For instance, England’s consumption of coal massively increased after James Watt introduced a new fuel-efficient engine that used much less coal in the late 18th century, as the lower operating cost made the engine viable for more use cases. If AI tools – still in their infancy in terms of development, deployment as well as usage – live up to the hopes of their acolytes, declining costs might lead to an increase in demand that outweighs the productivity gains. This would result in a need for more GPUs, not fewer.
While AI has been a significant driver of sentiment for many companies, for many semiconductor firms it represents a comparatively small proportion of revenues due to the relatively low volumes of GPUs manufactured. Some semiconductor firms, such as ASML, are probably more dependent on continued demand for high performance semiconductors for consumer devices than they are for AI demand – Nvidia likely sold around four million GPUs last year, but Apple alone probably sold 50x as many iPhones, each of which includes chips made with cutting-edge ASML tools. As a result, the earnings of some semiconductor firms are probably more dependent on demand for high performance semiconductors for consumer devices than they are on demand for GPUs.
While DeepSeek and lower cost ways to build LLMs are both worth monitoring, the capex indications of the hyperscalers are clear, at least in the short term, and it seems likely they will increase this by a further 20%+ this year. Microsoft and Meta report on Wednesday, and we would expect them to confirm their capex guidance. While news flow around DeepSeek may continue, we don’t believe it will ultimately reduce the demand for AI or GPUs. So long as we remain in a race to artificial general intelligence, both hardware and software improvements will likely be leveraged as quickly as possible.
Furthermore, we are in the early stages of AI – a long term transformative trend – and innovation is happening at a rapid pace. Claims of sudden or revolutionary advancements in this nascent field are likely to continue for the foreseeable future – similar to what we have seen over the past 18 months. This ties in with a key part of our investment philosophy: at the early stages of new technology development, it is normal to see standards changing or performance improving persistently – one must thus be very selective about gaining exposure to a new technology during a period when it is more challenging to identify the long-term winners.